AI Success Secrets: Strategies for Effective Project Management
Artificial Intelligence (AI) is revolutionizing the way businesses operate, offering unprecedented opportunities for growth and efficiency. As organizations increasingly integrate AI into their operations, effective project management becomes crucial to harness the full potential of AI technologies. Understanding the strategies for successful AI project management can significantly impact the outcomes of these initiatives.
Effective AI project management begins with a clear understanding of the project’s goals and objectives. It is essential to define what success looks like for the project and how AI can help achieve these goals. This clarity helps in aligning the project with the overall business strategy, ensuring that the AI initiative adds value and drives competitive advantage.
Another critical aspect of AI project management is assembling the right team. AI projects require a mix of skills, including data science, software engineering, project management, and domain-specific knowledge. Building a team with complementary skills and a shared vision for the project is vital for its success.
Project managers must also focus on data management, an essential component of any AI project. This involves ensuring the availability, quality, and security of data used to train and test AI models. Effective data management practices help in avoiding common pitfalls such as data bias and underfitting, which can derail AI projects.
Moreover, stakeholder engagement is crucial in AI project management. Regular communication with stakeholders, including project sponsors, end-users, and IT staff, ensures that everyone is on the same page and that the project meets the needs of all parties involved. This engagement also helps in managing expectations and fostering a culture of innovation and collaboration.
Adapting project management methodologies to fit AI projects is another strategic approach. Traditional project management methods may not always suit the iterative and experimental nature of AI projects. Agile methodologies, which emphasize flexibility and iterative progress, can be more effective in managing AI initiatives.
Risk management is also a significant consideration in AI project management. AI projects come with their unique set of risks, including technical uncertainties, data privacy issues, and ethical concerns. Identifying these risks early and developing mitigation strategies is crucial for the smooth execution of AI projects.
Continuous learning and improvement are vital in AI project management. AI technologies are rapidly evolving, and project managers need to stay updated with the latest developments and best practices. This not only helps in managing current projects more effectively but also in planning future AI initiatives.
Finally, measuring the performance and impact of AI projects is essential for validating their success and learning from their outcomes. This involves setting up metrics and KPIs to track the performance of AI systems and using these insights to refine and improve future projects.
In conclusion, effective project management is critical to the success of AI initiatives. By focusing on these strategies, organizations can maximize the benefits of AI technologies and ensure that their AI projects are successful and deliver tangible business value.
Key Roles in an AI Project Team
Effective AI project management requires a diverse set of skills and expertise. The composition of an AI project team can significantly influence the project’s success. Here are the key roles that are crucial for any AI project:
- Project Manager: Oversees the project’s progress, ensures it aligns with business goals, and manages resources.
- Data Scientist: Responsible for designing and developing algorithms, as well as data analysis and modeling.
- AI Engineer: Focuses on building AI models and integrating them into business processes.
- Data Engineer: Ensures the architecture and infrastructure for data collection, storage, and analysis are optimal.
- Business Analyst: Bridges the gap between AI capabilities and business needs, ensuring the AI solutions meet business objectives.
- UX/UI Designer: Designs the interface and experience to ensure the AI system is user-friendly.
- Quality Assurance Engineer: Tests and ensures that the AI system meets the required standards and functions correctly.
Each member of the AI project team plays a unique role, contributing to the project’s overall success. Effective collaboration and communication among these roles are crucial. Project managers should foster an environment where team members can share insights and learn from each other, enhancing the project’s innovative potential.
Data Quality and Security in AI Projects
Ensuring data quality and security is paramount in AI projects. Poor data quality can lead to inaccurate AI models, while data security breaches can have severe legal and reputational consequences. Here are strategies to ensure data quality and security:
- Data Collection: Use reliable sources and ensure that the data collected is relevant and unbiased.
- Data Cleaning: Regularly clean data to remove inaccuracies and inconsistencies.
- Data Protection: Implement robust security measures to protect data from unauthorized access and breaches.
- Data Compliance: Ensure compliance with relevant data protection regulations, such as GDPR.
- Data Monitoring: Continuously monitor data quality and security to identify and address issues promptly.
By prioritizing data quality and security, AI projects can not only achieve better outcomes but also build trust with stakeholders and users.
Common Challenges in AI Project Management
AI project management involves unique challenges that can impact the success of the project. Understanding these challenges is the first step in mitigating them. Here are some of the most common challenges:
- Scope Creep: AI projects can evolve rapidly, sometimes leading to changes in project scope. This can cause delays and budget overruns.
- Data Issues: Issues such as data scarcity, poor quality, or bias can derail AI projects.
- Stakeholder Alignment: Misalignment between different stakeholders can lead to conflicts and misunderstandings.
- Technical Complexity: AI technologies can be complex, and integrating them into existing systems can be challenging.
- Change Management: Introducing AI can require significant changes in business processes and culture, which can be resistant to change.
Addressing these challenges requires a proactive approach and a flexible project management strategy that can adapt to the evolving nature of AI projects.
Adapting Agile Methodologies for AI Projects
Agile methodologies, known for their flexibility and iterative approach, are particularly well-suited for AI projects. Adapting Agile practices can help manage the uncertainty and rapid changes often associated with AI initiatives. Here are ways to adapt Agile methodologies for AI projects:
- Sprints: Use short development cycles (sprints) to rapidly produce workable AI models and iterate based on feedback.
- Scrum Meetings: Regular scrum meetings can help keep the team aligned and quickly address any issues or changes in the project.
- User Stories: Develop user stories to better understand how AI solutions will solve real user problems.
- Retrospectives: Regular retrospectives can help the team reflect on what worked and what didn’t, continuously improving the process.
- Backlog Grooming: Continuously update the project backlog to prioritize tasks that deliver the most value.
By incorporating Agile methodologies, AI projects can be more adaptive and responsive to changes, ultimately leading to more successful outcomes.
Best Practices for Stakeholder Engagement in AI Projects
Stakeholder engagement is crucial for the success of AI projects. Effective engagement ensures that the project has the necessary support and that the solutions developed meet the needs of all stakeholders. Here are best practices for engaging stakeholders in AI projects:
- Identify Stakeholders: Clearly identify all stakeholders involved in the project, including end-users, IT staff, and senior management.
- Communication: Establish clear and open channels of communication with stakeholders to keep them informed and involved.
- Feedback Loops: Implement regular feedback loops to gather insights and suggestions from stakeholders, and adjust the project accordingly.
- Training and Education: Provide training and educational resources to help stakeholders understand AI technologies and their impact.
- Change Management: Develop a change management strategy to help stakeholders adapt to changes brought about by AI implementations.
Engaging stakeholders effectively not only helps in aligning the project with the needs of the organization but also builds trust and support for the AI initiative.
Measuring the Success of an AI Project
Measuring the success of an AI project is essential for determining its impact and value to the organization. It also provides critical insights that can be used to improve future AI initiatives. Here are key metrics and approaches to measure the success of an AI project:
- Performance Metrics: Define specific performance metrics that the AI solution should achieve, such as accuracy, speed, or cost reduction.
- User Satisfaction: Measure user satisfaction to ensure the AI solution meets user needs and expectations.
- ROI: Calculate the return on investment to assess the financial benefits of the AI project compared to its costs.
- Operational Efficiency: Evaluate improvements in operational efficiency and productivity resulting from the AI implementation.
- Innovation Impact: Assess how the AI project has contributed to fostering innovation within the organization.
By using these metrics, organizations can gain a comprehensive understanding of the success of their AI projects and identify areas for improvement.
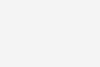
Ethical Considerations in AI Project Management
AI projects raise important ethical considerations that need to be addressed to ensure the responsible use of AI technologies. Ethical management of AI projects involves several key aspects:
- Transparency: Maintain transparency in how AI models make decisions, especially in critical applications.
- Privacy: Protect user privacy by implementing strong data protection measures and ensuring data is used ethically.
- Fairness: Monitor AI systems for bias and take steps to ensure they operate fairly and do not perpetuate inequalities.
- Accountability: Establish clear accountability for AI decisions, ensuring that there are mechanisms in place to address any issues that arise.
- Collaboration: Work with other organizations, regulators, and stakeholders to develop standards and best practices for ethical AI.
Addressing these ethical considerations is crucial for building trust and ensuring the sustainable and responsible deployment of AI technologies.
Conclusion
AI project management is a complex but rewarding endeavor that requires a strategic approach tailored to the unique challenges and opportunities presented by AI technologies. By focusing on key areas such as team composition, data management, stakeholder engagement, and ethical considerations, organizations can enhance their AI initiatives. Effective project management not only drives the success of AI projects but also helps in integrating AI into the broader organizational fabric, paving the way for transformation and innovation.
As AI continues to evolve, staying informed about the latest trends, challenges, and best practices in AI project management will be crucial. Organizations that can adeptly manage their AI projects will be better positioned to capitalize on the transformative powers of AI, turning potential disruptions into substantial competitive advantages.
Ultimately, the success of AI projects hinges on a balanced approach that considers technical, business, and ethical dimensions. By fostering a culture that embraces continuous learning and improvement, organizations can navigate the complexities of AI project management and achieve outcomes that are not only successful but also aligned with their strategic goals and values.
For those looking to dive deeper into specific aspects of AI project management, resources such as using a staffing agency like icreatives staffing, and ways to recognize employees provide valuable insights into effectively managing human resources in AI-driven projects. Additionally, exploring topics like boosting productivity with AI tools can offer practical tips for enhancing project outcomes.
As AI reshapes industries, the role of effective project management in leveraging AI for business success cannot be overstated. It is an exciting time for businesses to embark on AI projects, and with the right strategies, they can navigate this dynamic landscape to foster innovation and growth.
In today’s competitive market, finding the right creative and marketing expert can be a challenge. But with icreatives, you’re in experienced hands. With 37 years in staffing and a track record of matching more than 10,000 employees to over 1,000 companies worldwide, we know how to connect you with the best. Plus, you only pay if you hire—there’s no risk, only results.
Ready to find your perfect creative or marketing expert? HIRE WITH ICREATIVES today!